Control Theory and Reinforcement Learning: Connections and Challenges
Control theory and reinforcement learning (RL) converge on a shared objective: facilitating autonomous, real-time decision-making to optimize dynamical processes. Historically, these disciplines have diverged in assumptions regarding available prior information and in analytical techniques applied. However, recent advances bridging the two domains are fostering collaborations. The CWI research semester programme in spring 2025, themed "Control Theory and Reinforcement Learning: Connections and Challenges", comprises a spring school and workshops on various sub-topics to explore the intersections and challenges within these intertwined fields.
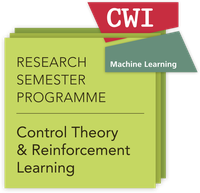
Spring School
This spring school emphasizes connections across control theory, reinforcement learning and stochastic approximation, enabling students to access these broader themes and start to work on cross-cutting projects. The school will be at a preparatory PhD level, suitable for advanced Master's and starting PhD students in these areas.
Programme
17 March | 18 March | 19 March | 20 March | 21 March |
---|---|---|---|---|
Registration: Welcome by |
Walk-in |
Walk-in |
Walk-in |
Walk-in |
Deterministic, discrete-time Bert Kappen |
Generalized policy Frans Oliehoek |
Stochastic Sean Meyn |
TD-learning & actor- Sean Meyn |
Multi-agent games: Maryam Kamgarpour |
Break |
Break |
Break |
Break |
Break |
Deterministic, discrete-time Bert Kappen & TAs 11:15 - 13:00 |
Generalized policy Frans Oliehoek & TAs 11:00 - 12:45 |
Stochastic Sean Meyn & TAs 11:00 - 12:45 |
Q&A on tutorials Lecturers & TAs 11:00 - 12:45 |
Multi-agent RL / Frans Oliehoek |
Lunch Break & Posters |
Lunch Break & Posters |
Lunch Break & Posters |
Lunch Break & Posters |
Lunch Break & Posters |
Stochastic optimal Bert Kappen |
Actors and Critics: Debabrota Basu |
Q-learning Sean Meyn |
Optimism (UCRL, PSRL, Debabrota Basu |
Panel Discussion Sean Meyn, 13:30 - 15:00 |
Break |
Break |
Break |
Break |
|
Stochastic optimal Bert Kappen & TAs 16:00 - 17:45 |
Actors and Critics: Debabrota Basu & TAs 16:00 - 17:45 |
Q-learning Sean Meyn & TAs 16:00 - 17:45 |
Safety in RL & control Maryam Kamgarpour |
|
Social |
Games evening |
Dinner |
||
Teaching Assistants (TAs): Caio Kalil Lauand, Guillaume Pourcel, Mustafa Mert Celikok, Rea Nkhumise.